Maintenance 4.0: is generative AI changing the game?
21 February 2024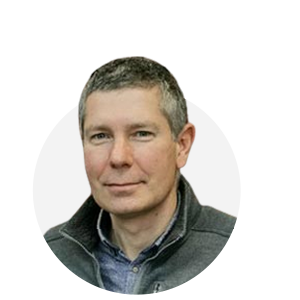
The famous ChatGPT conversational agent is still making waves! And with good reason: Large Language Models (LLMs) have recently come to the attention of the general public thanks to their incredible performance on human-like tasks (dialogue, text, image or music generation, etc.) and have rapidly become the most visible models on the generative AI iceberg.
If certain use cases are now affecting the industrial sector, what is the situation in the field of industrial maintenance? Will generative AI transform maintenance jobs?
Interview with Christophe BIERNACKI, Inria’s Deputy Scientific Director for Applied Mathematics.
AI AS A LEVER FOR OPERATIONAL PERFORMANCE
Given the popularity of generative methods, particularly those based on deep learning, there is a strong temptation to apply these technologies to other fields, including the industrial sector, and in particular predictive maintenance. This is confirmed by Christophe BIERNACKI:
“In this context, a useful generative mechanism would then produce a prediction of the future state of an industrial system over a given time horizon, or even ideally a precise recommendation to prevent a failure from actually occurring. The promise is tantalising. ”

But let’s not confuse AI with generative AI. While AI is a general term for the implementation of methods to enable machines to imitate a real form of intelligence, generative AI is a sub-branch capable of generating text, images or other media.
The maintenance sector has been revolutionised by AI, particularly with predictive maintenance. By analysing the health of industrial equipment in real time, predictive maintenance goes beyond the limits of preventive and corrective maintenance. Its strengths? Detecting patterns that herald malfunctions from a variety of data (functional, categorical, incomplete, etc.), even when there are few examples of failures in the historical data.
A very different approach to generative AI, which requires a large learning corpus.
“In the context of predictive maintenance, it is well known that the most important data, i.e. that recording a significant history of failures, is present in a particularly small number. It is therefore illusory to successfully apply current generative methods in this precise context, with more standard machine learning or statistical techniques still largely to be preferred.”
We must also pay the greatest attention to the robustness of these generative methods in the maintenance sector when these prerequisites are not met:
“To be effective, generative methods need to learn from large quantities of data representing phenomena close to those to be generated later. Generative AI, and LLMs in particular, suffer from a number of limitations and raise a large number of associated risks.
The biggest risk comes from the lack of reliability and robustness of these models: it is now well known that they regularly produce hallucinations, i.e. state as true results that are trivially false, but perfectly plausible for the neophyte in the field concerned. This type of error could of course be limited by access to reliable sources of information during inference.”
BUT WHAT ROLE CAN GENERATIVE IA PLAY IN MAINTENANCE?
In practical terms, generative AI can now be used to support manufacturers in their HR challenges, particularly in the area of team leadership. According to Joël Thibert, project director at McKinsey in Calgary, in an article published in January in les Echos, generative AI applies to the drafting of maintenance reports and the transmission of knowledge by creating an operating range with detailed instructions, automatically synthesising technical manuals. This will help prevent the loss of knowledge in a sector that is struggling to recruit.
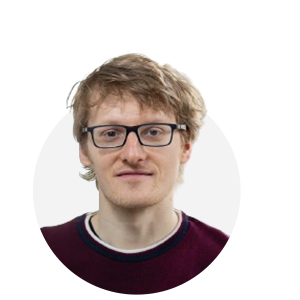
As you can see, generative AI can help teams save time on known, repetitive tasks, but it is not suitable for monitoring machines, as our Head of Data Science Quentin GRIMONPREZ explains:
“In order to be effective, AI techniques must meet the specific needs and challenges of the industrial context: few fault histories available, variety of equipment usage contexts, data from multiple sources, in multiple formats, of different natures and/or with a greater or lesser degree of certainty, etc.
While some faults are recurrent and well-known, many are new! So we need to be able to deploy robust Artificial Intelligence solutions capable of identifying new abnormal behaviour and diagnosing new types of malfunction”